The Hidden Power of AI and A/B Testing: Lessons in Experimentation at Scale
What makes experimentation and A/B testing essential for improving user experiences, and how does AI take it to the next level? In this episode of AI Experience, Collin Crowell, VP of Growth at Kameleoon North America, shares his insights on building robust experimentation programs and using AI to make smarter, faster decisions. You'll learn how A/B testing provides the data you need to improve your digital products and why collaboration across teams is crucial for scaling experimentation. Collin also explores how AI can help you identify opportunities, optimize targeting, and streamline workflows—without losing sight of the human touch. If you're looking to take your experimentation strategies to the next level, this episode is for you.
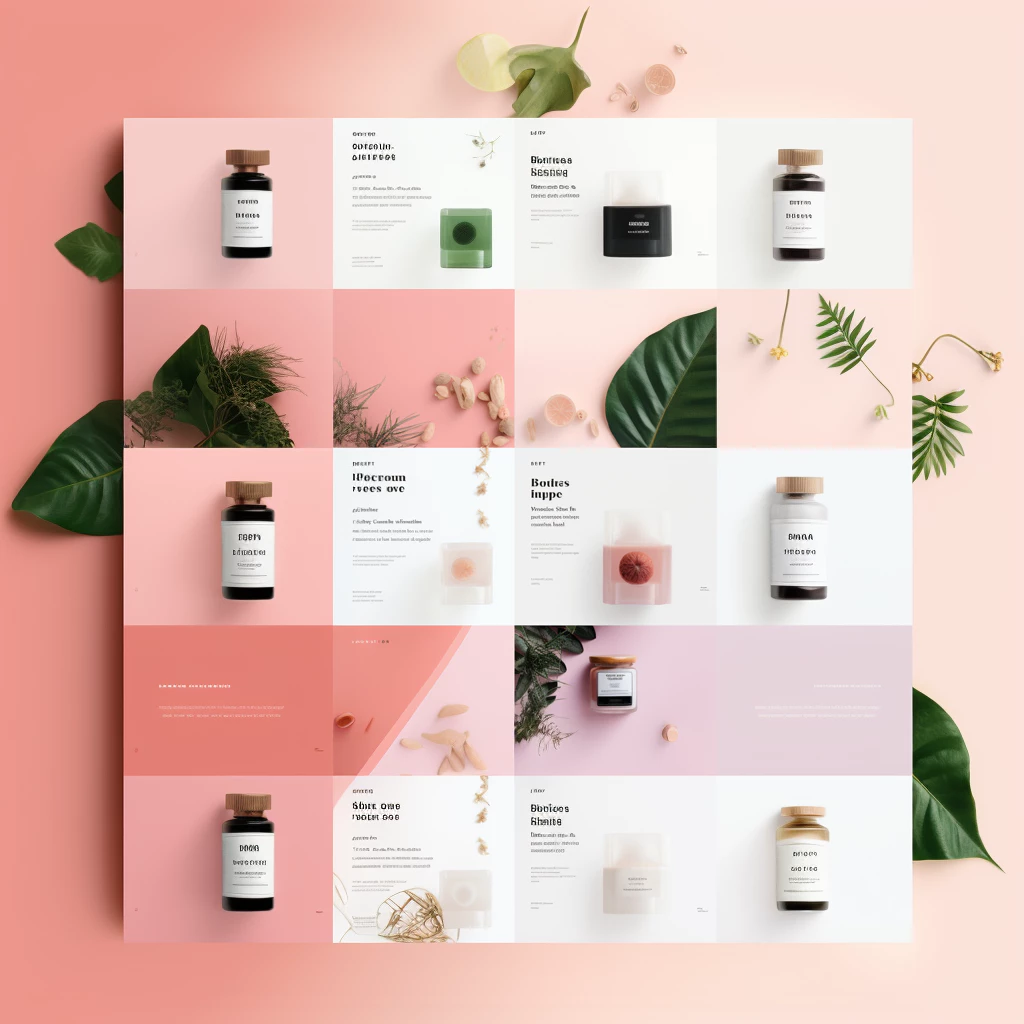
Collin Crowell is VP of Growth for Kameleoon, North America, leading sales, marketing, and partnerships for the region. Prior to joining Kameleoon, Collin helped build GO Group Digital, a transatlantic consultancy dedicated to providing international experimentation services. His international work includes acting as digital publishing lead in China and Southeast Asia for Ringier AG, a Swiss media company. Today, he is based near Whistler, Canada where he can ski and mountain bike.

Collin Crowell
VP, North America
Julien Redelsperger: "And I'm super happy to welcome Collin Crowell. He's the VP of Growth for Cameleon North America, leading sales, marketing, and partnerships for the region. Today, we will talk about AI, A/B testing, and experimentation. Thank you for joining me today. How are you, Collin?"
Collin Crowell: "Thank you very much for having me. I'm doing well today."
Julien Redelsperger: "So for those who are not familiar with experimentation and A/B testing, let's probably get back to basics for a few minutes. Could you explain to us what it is and how is it useful?"
Collin Crowell: "Yeah, happy to. I remember somebody asked me the same question and I heard another person give a better answer than this. And I'll just say it. A/B testing, at the end of the day, uses math to tell you why your digital products aren't as good as you think. And let me unpack that a little bit for you. Essentially, A/B testing, or experimentation, or conversion rate optimization, it has many names. But essentially, at the end of the day, what you're doing is you're using the scientific method to build better customer experiences or build better digital products. And what I mean by that is you can understand a customer problem and create a hypothesis on how to fix that customer problem and then you can test a variation of the current way to solve that problem against a variant. So if a customer's having a problem checking out, for example, of your e-commerce site, you could propose a new way to check out and you could compare the two, comparing the control and the variant to see which one helps the customer check out. And when you do this at scale, essentially statistics give you insight into which version is better for the vast majority of customers. And that's really what A/B testing is at the end of the day. It's giving you proof what is working for your customers or not. And based off that proof, the teams, managers, they can make more informed decisions about how they wanna grow their business or what do they wanna do with their company."
Julien Redelsperger: "And so you just mentioned e-commerce. So who are your clients in North America? Can you share some names?"
Collin Crowell: "Yeah, so we work across five different verticals primarily. One of the biggest verticals is e-commerce, but interestingly here in North America, we do a lot of work with banks and US healthcare companies. So examples of our customer portfolio would include famous e-commerce brands or retailers like Canada Goose, huge groups of fashion that you've never heard of. I'm sure you've heard of the Fossil brand, but that entire group includes like Diesel and DKNY. So large e-commerce or fashion brands. But then when you move over to the banking space, you're gonna see a lot of FinTech companies. And for example, here in North America, we'll be working with large hospital groups like Providence Healthcare. So yeah, we do most of our business in those spaces."
Julien Redelsperger: "Okay, and so I understand when you run a single experimentation, page A, page B, which one is the best? It's pretty easy to do, but I think the main challenge is building a robust experimentation program. Could you explain a little bit what it means and where does Kameleoon step in to help?"
Collin Crowell: "Essentially building the test at the end of the day, it turns out, it was relatively easy. In fact, there's lots of different parts to the process. Sometimes a lot of people will just look at testing like splitting traffic. To skip and make this simple, I don't think the build part or the splitting traffic is that hard. What's really hard to do is to make sure that you're getting high quality data back. And I think that's something that a lot of teams overlook, especially in the beginning, is they're so fixated on how do I make it easy to build a test that they forget that the whole purpose of A/B testing or experimentation is that you get more quality information about what your customers want faster than your competitors do, and then you're able to make more informed decisions. And so I think when I hear, how does Kameleoon help companies or teams build a robust experimentation program? I'm really trying to look at it through that lens, which is how do we help the different teams that should be involved in experimentation build tests, 'cause there's different ways to build a test. And then how do we make sure that those teams are able to use the technologies that are important to them in their day-to-day work? And then finally, how do we verify and give them confidence that the decisions they're going to make from the outcome of those tests are credible? And if I think if you can reassure and prove to various teams those three points, then you allow a company to have, essentially unlock the capability to build a robust test and learn program or an experimentation program. But if you don't do those three things, then I think you're in big trouble. So that's a long-winded way of saying Kameleoon's unique in that it's going to give every single team the ability they need to build the test, to use the technologies that are important to them, and to use the statistical methods that they think are helpful to analyze their tests. And to go one step further, Kameleoon's unique in what we call all-team experimentation. All-team experimentation not only refers to, of course, letting all the teams participate in it, it's also making sure that those teams understand the impact that their work is having on another team. And I think that's where other testing tools fall short. One of the reasons I liked Kameleoon and wanna grow Kameleoon here in North America is 'cause I believe in that technological vision, which is it's not just that you have to give marketers a graphic editor to build a test without any code, or it's not just that you have to give engineers the ability to build a test using a feature flag or built on an SDK. That's important, but more important is that, does the marketer understand how they're optimizing the customer journey affect the product downstream? For example, I heard this great, we were at experiment, every year we'd build something called EXL Unite. And we had somebody from Amazon there at one of our tables, and they were talking about how they're able to see how a promotion by a marketer is affecting the listening time of a subscriber later on. And I think that's a really good insight that I hope a lot of people think about as they explore building a test and learn program is how well can you, how easy can you make it to build a test? But more importantly, how well can you see how your work is affecting the entire customer journey? Because this is what leaders really do compared to like newbies or laggards, or if you really wanna go from good to great, you gotta figure that out."
Julien Redelsperger: "Okay, and so A/B testing has been existing for a while, maybe 15 years, 20 years. Do you need AI to run A/B tests?"
Collin Crowell: "No, absolutely not. You don't need AI. I think that's the big distinction I'll make is that I don't think you need it. But just like a lot of different functions at a company, there's a lot of tedious manual work that is part of, it can be part of experimentation. And I think currently AI is really good at stepping in and helping teams eliminate the amount of time they're doing for tedious, repetitive tasks. And this is one example that I'll just share with you and your audiences. The amount of time it takes, remember, let's go back to the scientific method, right? We're trying to, where are the problems, okay? If you're not really identifying a customer problem, you're in big trouble. And I think AI is helpful there right away. So you can apply AI to look at all of your reviews on, like let's say your SaaS tool, and or, you know, let's get back to e-commerce. Let's imagine you're an e-commerce brand. You can look at hundreds, thousands of different reviews by customers about your product, and AI will very easily surface customer problems. And that's a starting point. You can find, you know, okay, what's a problem that if I fixed would improve the overall customer experience? And I think AI is incredibly good at that compared to the amount of time it would take, the amount of money it would take to hire focus groups and go or hire a research company and go through all of those customer reviews. Well, now we know AI can do that in a matter of minutes."****
Julien Redelsperger: "Okay, so what I understand is that we don't need AI to run tests, but Al makes it better, faster, stronger. That's what you're trying to tell us."
Collin Crowell: "I think AI, like I said, is really good at automation. And I think it's incredibly, I particularly believe it's incredibly good at being creative at a scale or pace than a human. So to cut to the chase here, I think Al is incredibly valuable and helpful to experimenters when it comes to a few things. Ideation, first of all. What should you be doing? What could you, I take that back. That's an important point that I'll take back. What could you be doing? I think Al is extremely strong at this point. It can surface in hundreds, if not thousands of suggestions because it's so good at ideating. Now, I said, I'll take back what should you be doing? I'm not convinced that AI is ready to tell you what you should be doing. And I think that's an important distinction that anyone interested in AI and experimentation needs to consider. There's a big difference between what you could do and what you should do."
Julien Redelsperger: "Okay, and so if AI can generate thousands of tests like in minutes, does that mean you should be testing everything or is there a risk of overwhelming companies with too many tests and too many decisions to make?"
Collin Crowell: "You know, I could give you the safe answer and tell you, it depends. But before I give you the safe answer, I'll just give you, I think, the answer you're expecting or the one that seems intuitive. And the answer is no. The idea that you, just simply because you could doesn't mean you should run thousands of tests upon commencing experimentation. A more nuanced reply would be to tell you, well, hold on. You remember what I said earlier, experimentation is about using math to tell you how your digital products and digital experiences are performing. You simply will not have enough statistics. Most, if not, I would say there's 0.001% of companies that could afford to run a thousand tests at a single time because they simply don't have enough statistical power. Their traffic to their sites is not large enough to give them the statistical proof that the variant they've created is actually having a positive impact."****
Julien Redelsperger: "So what would be- What would be the minimum threshold to run statistically significant tests?"
Collin Crowell: "Gosh, there's entire calculators that are designed. And again, the hard answer is it depends. So there's some cases where if the, like for example, the number of users could be quite low, but if the conversion rate is quite high, then you don't need that many users into the test. But for the sake of today's discussion, I think you, and I'll just tell you here at Kameleoon, we try to work with companies that have a minimum of 250,000, what we call monthly unique users coming to their site or to their app, for example. And I think that's a useful way just to kind of ballpark, what are we talking about here? So a lot of companies, young companies may be interested in experimentation, but if you have 50,000 people coming, well, first of all, congratulations, you got 50,000 people to come to your site every month, but it's still, experimentation still may not be the best bang for your buck, just because you don't have that statistical power to run one experiment, much less thousands of them."
Julien Redelsperger: "Why are you excited about experimentation, Colin? What do you like about it?"
Collin Crowell: "It's like having a crystal ball. If I, and this is what's so amazing, I told you about the event that we organize every year, it's called EXL Unite or Experimentation Unite. And we're lucky to have a chance to connect with hundreds of different practitioners, experimentation practitioners, huge companies. And what's remarkable is that all of these practitioners really are so frustrated with the adoption of experimentation at their company. And I'm always curious as to why, what's stopping this? Because it's crazy when you think about it. If you went to your boss and you said, hey, I can tell you, I can give you the future. I can give you this crystal ball and you can look inside it and you can see what is actually having a positive impact on your KPIs, whatever you think is important. And you can see that not only in real time, but you'll see it faster than your competitors can see it. And you're telling me you don't want that? You don't want to see this applied across your entire organization? This to me is bewildering because it's just so powerful. And I think that power is what attracts me to experimentation is that you have this incredible capability to see what's truly satisfying your target customer or your target user."
Julien Redelsperger: "And AI could improve that power."
Collin Crowell: "Absolutely. So again, we look at here at AI and experimentation in four different ways. Well, first of all, it has to affect the entire experimentation process or workflow. And so we break down that workflow into more or less like four parts. So part one, as I mentioned, is about ideation. So here we call that Al experiments. And then part two is targeting. So one of the more interesting ways that you can apply AI is to predict on different segments where your new digital experience will have an improvement or not. So for example, it's really impressive how you can use Kameleoon AI to say, listen, I'm trying to improve this KPI. I want you to target all users who will positively react to this experience to boost this KPI. And that's what we call predictive targeting. And again, it's remarkable. The e-commerce companies, this is a classic example, is they're often given like 10% off to everybody. Sign up for my newsletter or check out right now and I'll give you 10% off. Well, with Al, you don't need to apply that 10% to everyone. AI can simply look at all of the different visitors coming to your site, watch their behavior, and essentially decide very effectively on who to give the 10% discount to because it doesn't look like they're going to convert. For those that are actually gonna check out, why would you give away 10, 15% of your margin? They're gonna check out anyway. So I think that's a really useful way of using Al for targeting purposes. And then there's times where an experimenter will build a test, they'll target a segment, and the test will lose. We call this a lose, or it doesn't win, I should say. It doesn't improve the KPI like you thought it would. Well, Al is, again, very good at looking at large quantities of data and telling the optimizer or the experimenter, listen, your test, you think it didn't work, and you're right, it didn't work for users Monday through Friday, but look at what happens to your performance on the weekend. Or look at what happens to the user experience when they move over to their mobile app. Or back to desktop, for example. Here, we call that detection, AI opportunity detection. And I think, again, that's a really powerful way of using Al to help you find value that is really hard for humans to find easily. And then the last one is what we call AI assist, and that leverages what I think a lot of people are familiar with, using Al or generative AI to have a conversation with, that's helpful. So you can ask a Kameleoon AI assist, what statistical method should I use here? Or how many days do I have left to run my test? Or can you summarize the key insights from the last three tests for me? Or how do you do this again? So I think that kind of general assistant is understated, but just as important as those other parts that I described."
Julien Redelsperger: "So when you talk to your clients, when you talk to companies or experts, do they say that AI is a key component of an AI, of an A/B testing platform?"
Collin Crowell: "Yes, I think honestly, you have to say that now about any software. I think if you're not thinking creatively about how to use Al in your work, then you're missing out. So that's just a general blanket statement, but even more so in what we call experience optimization or digital experimentation. Here where you're dealing with digital experiences and large amounts of data and complex environments, I think AI is a must. I think anyone looking at it though, has to be really thoughtful because if everyone's throwing out the word AI and AR, you really need to look at, okay, what's their credibility? What's their history? What's the application? And you need to spend a lot of human time vetting that the AI is in fact, not just a gimmick, but something quite substantial.
Julien Redelsperger: “So we can't really talk about AI and A/B testing if we don't talk about data. And we know that sometimes in organization, it could be chaotic, messy. And how does that limit AI's effectiveness to be like a smart assistant? How do you manage data IGM to make sure that AI could be effective and A/B testing as well?"
Collin Crowell: "Yeah, it's a good point. You know, a couple of things that we have to think about here is one, like a puppy, AI is eager to please you. And sometimes this is referred to as hallucinations, but it's, unless you're very careful with AI, it's able, or maybe it's too eager to please and it will ideate irresponsibly or it can go beyond, I think, the risks that the operators wanted to. And so I think that's one thing is that in the absence of good data or in the absence of high cleaned data, AI takes risks that I'm not sure humans are comfortable with. So that's point number one. But point number two, I think is, you know, what we're all more or less faced with today, which is garbage in, garbage out. So how effective can Al be if you don't have data hygiene? So that's where I think most companies, and we're part of that too, I don't wanna pretend like we figured it all out here at Kameleoon, but recognizing that if you really want AI to deliver value, you first need to make sure that you have great data governance, data hygiene, because until you have that, you really are not capitalizing on the value of AI. And then, like I said, you're risking that Al is going to ideate irresponsibly. And that's why it's so important to get your data hygiene right."
Julien Redelsperger: "Did you see some change or evolution in the job market when we talk about A/B testing and experimentation? My question is, how is the skillset evolving for today's experimentation leaders? Can you be an experimentation leader if you don't know much about AI, or do you need to be fluent in AI to be a good experimentation person, professional?"
Collin Crowell: "Yeah, I think it is incumbent upon anyone that's looking to build a career in experimentation that they demonstrate robust competency in AI. What that means and how you present it, I'm not the greatest judge on this. I just would point out that it would be silly for anyone getting into digital marketing or any digital space to not be taking advantage of this incredible tool that is still at relatively early stages. So I think what you're looking for is someone that's, again, very curious, very data aware, and always eager to find new ways and how they can simplify and automate things using any tool, but particularly now, AI. So yeah, I think, yeah, if anyone's looking at a career in experimentation or looking to grow their career in experimentation, absolutely need to look hard at AI. And then those that are, or have experience and have proof points that they can bring in job interviews or talk about in meetings, wow, yeah, I think that's gonna be a really sought after person."
Julien Redelsperger: "How do you keep up with Al news? I mean, every day, every week, we have new features, new information, new LLMs, models, tools, platforms. It could be overwhelming sometimes. How do you deal with that?"
Collin Crowell: "I wish I had the solution to tell your audience. There is so much. So again, I think you have to be really, like for me, I'm interested in building a great Kameleoon in North America. And so I'm looking at different, how Al is used in experimentation. And I think because I'm looking at it in this clear vertical, in this clear industry, that it minimizes all the noise there is around AI overall. So just by staying focused on where it may be most helpful could make it easier for you to stay on top of it. Give you some examples. I subscribe to, of course, all experimentation news. And again, everyone's so captivated by what's happening in the AI space, that often Al is in these media outlets, and that's how I stay on top of it. And I gotta say, I listen to podcasts like yours."
Julien Redelsperger: "Thank you. And so you mentioned that you were recently at the Excel Unite event in Austin, Texas, which is one of the most important events in the experimentation world. What did you learn from this event? And did companies and attendees talk about artificial intelligence in the experimentation world?"
Collin Crowell: "Well, we touched on the two topics that you've already raised, which was how important it is to understand the data. I think what we're seeing is just the abundance of data, right, and that can bring great value, but it can also bring a lot of headaches. And I think that was a key theme at this year's Excel Unite, teams beginning to be more aware of the importance of data. And then the, and of course, AI. I mean, every single table we were at, every single talk, the word AI, in fact, I think you were penalized if you didn't bring up the word AI at Excel Unite. So again, it's part of the conversation already, but let me give you one thing that I was impressed with that connects the two, AI and data. I noticed that there were a lot of teams that had not made that leap from building, many of them are good at, teams are good at, like, for example, the workflows and the processes to build a robust and rigorous experiment. And some of them have begun to create insight libraries, like databases, what they've learned through experimentation. Most of them, the few that have done that, however, have not easily begun to use Al to help query those databases to generate more profound insight. And I think that was something that I remember thinking was exciting. I look at it optimistically, like, wow, I bet if we go back next year, we're going to see a lot of advancement in that particular space. Because what's gonna happen to me, to get back to your question of data and what we've learned at the Excel Unite, is that we're going to be able to hopefully connect more data sources, clean data sources to one another. And once that data is in a place where it can be responsibly queried, then you're going to see Al to get better at what should you do versus what could you do. One of the things that we ask different executives is a question like AI prompts for the future. Things you could ask it tomorrow that you can't ask it today. And one of the interesting ones I remember hearing was, what tests should I build that will have the biggest impact on shareholder value in quarter, whatever, one? Wow, all right, that was interesting. What tests should I prioritize based on my CEO's presence on Bloomberg last night? Oh, geez, okay. Now that amount, to be able to ideate based on that query responsibly again, means connecting so many different data sources. And to me, that's really what Excel Unite began to explore, but still has room for development."
Julien Redelsperger: "Do you think that in the future, we could see fully 100% AI automated experimentation program with no human in the loop? Like you say, hey, artificial intelligence, this is my problem, you gotta run my experimentation program to make sure I can solve it."
Collin Crowell: "Yeah, I mean, again, I'll never say never, never say always, right? So I think it's possible. I don't think we're anywhere near that phase. I think there's a long ways to go. I would also add that AI is, and again, any good AI will tell you, I too do not know, and so let's test. And I think the bigger bets or bigger, the wilder, more different, the variations the AI creates, the AI should be more vocal about the importance of testing it. So I never think that any experimentation should be run in autonomy without human oversight. And I never think that any AI that's involved in experimentation would back off from experimentation. If anything, they're gonna wanna lean into it more and more. So the day could come, but just because you have the ability to use AI for testing doesn't mean that you shouldn't test even more aggressively.
Julien Redelsperger: "Okay, what's the main difference between experimentation tool like Kameleoon and in-house experimentation tool that big companies sometimes create themselves? Do you think that those in-house tool might have an Al component as well? And how do they manage it?"
Collin Crowell: "Well, again, I can't tell you how many times, every single day we are contacted by a company, by someone at a company who is quietly contacting us with this whisper in their voice to say, 'Please help me.' A few years ago, my company built this A/B testing tool in-house and no one's touched it since. It's miserable. I can't believe we still have to use it. And I'm inquiring whether or not you could help us replace the in-house tool with a Kameleoon or another tool. So point number one is A/B testing technology has advanced so far that I do not think it makes sense for most companies, I mean 99% of them to build an in-house testing tool. Are there some that make sense to build an in-house testing tool? Of course. Should big companies that are extremely mature in testing build an in-house tool? Absolutely. And I'm referring to say like a Netflix, an Airbnb, an Uber. These companies really have gotten to a place now where testing is so robust and rigorous across all channels, all parts of the customer journey that an in-house tool may make sense for them. In those situations, I also believe that they are relying on AI for all the things that I described earlier, which is to help with the experimentation process. But also I think to look at the data through a more powerful lens, which is less about what could you test and more about what should you test. And I think that that's what's most exciting. So I don't think the AI is any better when it comes to like targeting or detection or even assisting. I do think that at a certain giant level where very mature companies have built test and learn programs, if those data sources are connected, just imagine the ability to like ideate tests. Like, hey, I'm looking, give me 10 tests that are going to increase the lifetime value of a customer. Well, hold on, you would need to know and have access to so much data, particularly the lifetime value and what contributes to the lifetime value in order to be able to rigorously propose hypotheses that would possibly help increase that lifetime value. And I think that's what more advanced companies are gonna be good at is connecting their data sources and using advanced AI to like generate more impactful ideas to test."
Julien Redelsperger: "Okay, well, thank you so much. Oh, maybe one last question for you before we go. When we talk about AI and A/B testing, we talk about data. When we talk about data, we talk about data privacy. Is it a big topic these days in the experimentation world when you plug an AI solution to an experimentation program? Because you don't really know where the data is going when it is managed by AI. Is that a concern or is it just business as usual and that's fine?"
Collin Crowell: "I think it's, first, it's a concern, but I don't think, I think the excitement around AI is outweighing the concern at this stage. But I bet, and from what I'm seeing, is that the transition is happening quickly. I think it's incumbent upon anybody to ask hard questions about how their data is being safeguarded. And I think you're gonna see that more and more. So for example, here at Kameleoon, data is quarantined, data is segregated. You have to like, why should a company, let's take a fashion company, why would they want their insights shared with other fashion companies? That's a competitive disadvantage. So I think you need, again, to see with your vendor, how are they properly handling data privacy and data safeguards, and how long have they had experience with AI in order to give you confidence and reassurance that they're addressing the problems that you raised."
Julien Redelsperger: "Okay, perfect. Well, thank you so much. So at the end of each episode, the guest must answer a question posed by the previous guest. After that, you'll have the opportunity to ask a question for the next guest. Are you ready?"
Collin Crowell: "I think so. Let's go."
Julien Redelsperger: "All right, so here's your question, courtesy of Daniel Marcous, who is the founder and CEO of April, which is the first AI-powered tax system to reinvent how Americans approach their taxes. We can listen to his question right now."
Daniel Marcous : "Given the recent and somewhat debatable uprise in the abilities of LLMs to really reason and truly start powering agents like GPT-01 model, what do you think is the next industry that can be disrupted now, given those improvements that couldn't have been disrupted a month ago?'"
Collin Crowell: "Well, it's a great question. Honored that I get a chance to ask it, or answer it, excuse me. Where can those agents have an impact on other verticals? My head is at a place where what comes to mind is that we're always looking at Al to solve some utilitarian issue, like filing my taxes. I think that's a great example. Or help me book a flight. And I think what would be interesting to look at, which I'm not sure we're quite there yet, is what does it mean to have a companion? And so I'm curious whether or not Al is going to disrupt the dating spaces, like the dating apps. And not just from a utilitarian place of pairing people to someone that may be of interest to them. I'm curious about what AI can mean to companionship and solving loneliness, and blurring the lines between giving you genuine feelings with a virtual technology. So to me, that's an area for disruption. Like if somebody could, and I think you're seeing this already, like virtual boyfriends and girlfriends or virtual dates. And I think there's some really interesting questions about are those real or not? Because the feelings and the value that the people seem to be getting from them are pure to be."
Julien Redelsperger: "Wow, thank you. So now, Collin, what question would you like to pose for the next guest?"
Collin Crowell: "I would like to know, what's a misconception in your work that you wish more people understood? And I'd love to know how would correcting that misconception change the way they approach the work?"
Julien Redelsperger: "Thank you. Collin, it's been an absolute pleasure speaking with you today. Thank you for joining me."
Collin Crowell: "Julien, thank you so much for having me. Pleasure to be with you."
This transcription was generated by an artificial intelligence tool. It may not be 100% accurate and could contain errors and approximations.