AI Unplugged: Demystifying the Tech That Shapes Our World
Are you curious about how artificial intelligence is transforming our daily lives and business landscapes? In this episode of AI Experience, I sit down with Sidney Shapiro, PhD, Assistant Professor of Business Analytics at the University of Lethbridge's Dhillon School of Business in Canada. With over a decade of experience in data science and AI, Sidney brings a unique perspective on making complex data accessible and actionable. Join us as we explore the current state of AI, its practical applications, and what the future holds. From the rise of generative AI like ChatGPT to the integration of AI in everyday devices, Sidney shares his insights on the opportunities and challenges that lie ahead. Whether you're a tech enthusiast or just starting to learn about AI, this episode will provide you with a deeper understanding of the technology that is reshaping our world.
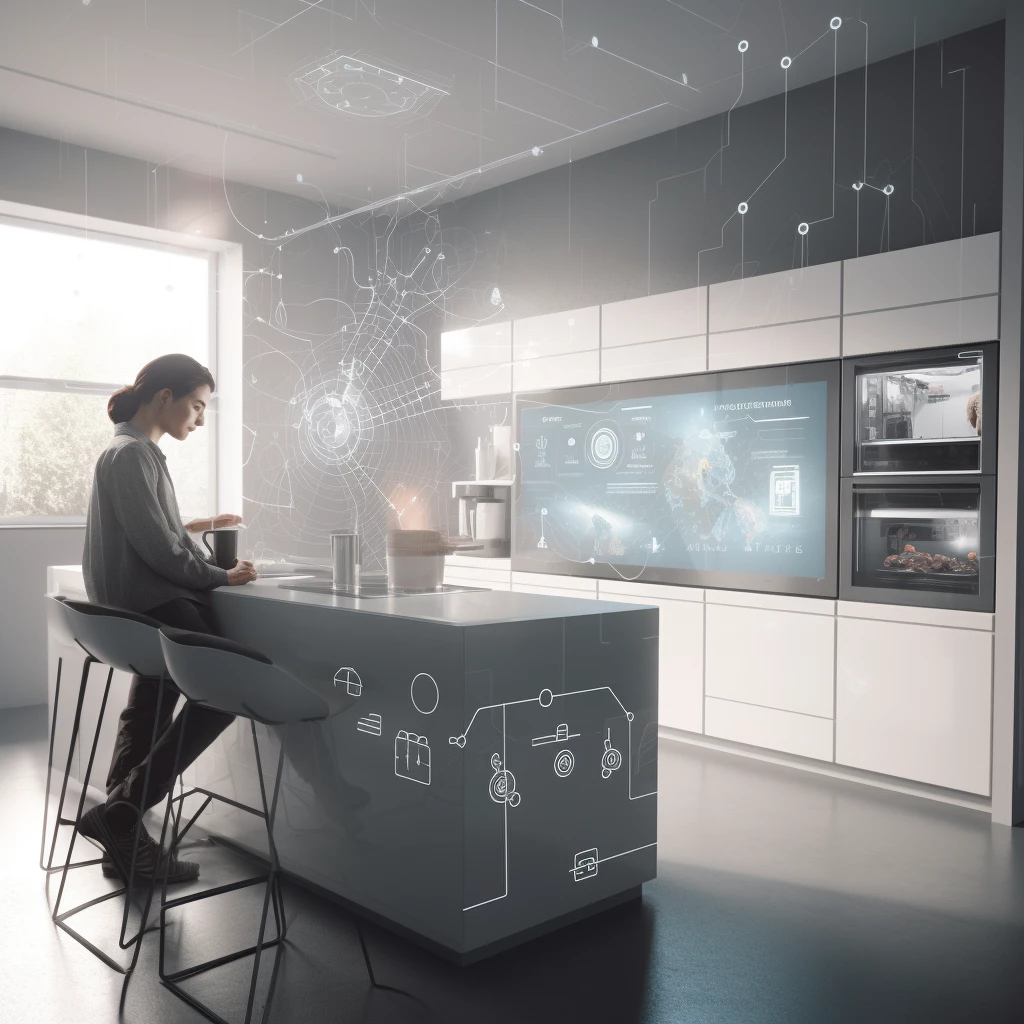
Sidney Shapiro, PhD, is a data professional with over a decade of experience in data collection, analysis, and storytelling. As an Assistant Professor of Business Analytics at the University of Lethbridge's Dhillon School of Business, he specializes in making complex data accessible and actionable, blending AI, data science, and business intelligence. Sidney's career includes roles as a data science Manager, where he led innovative solutions in occupational health and safety, and as a consultant, where he bridged research, business, and computer science to enhance operations and drive growth. Sidney's research focuses on AI adoption, program evaluation, business data transformation, and practical AI applications across industries. He holds a PhD in Multidisciplinary Studies - Social Network Analysis and a Master's in Applied Social Research from Laurentian University.

Sidney Shapiro
Assistant Professor of Business Analytics
Julien Redelsperger : « I'm super happy to welcome Sidney Shapiro. He's the assistant professor of business analytics at the University of Lethbridge-Dillon School of Business in Canada. Today we'll discuss the future of AI to understand the direction of this technology that surprised many organizations and individuals less than two years ago. Thank you for joining me, Sidney. How are you today? »
Sidney Shapiro : « Fantastic. Thank you, Julien. »
Julien Redelsperger : « It's my pleasure. We're going to have a great conversation about the future of AI. My first question is simple. Why now is a good time to know more about artificial intelligence? More importantly, is it already too late? »
Sidney Shapiro : « I think it's never too late. I think we're right at the very beginning of an amazing new technology. Over the past couple of years, we've seen a huge acceleration in software and what software can do, but we haven't yet seen the same type of thing happen with hardware. Once those two come together, we're going to be able to build new products that have AI built into them. For example, like a fridge or a microwave that can also understand what you want, that can do special programming and special things. We're right at the very, very beginning of that. The idea of getting into AI now, we're right at the very beginning. Literally, every day something new happens. I would say it's the perfect time to start learning about it because in five or 10 years, it will be very different. Yeah, because AI is not new per se, like people in universities and labs have been working on it for the past 50, 60 years. »
Julien Redelsperger : « When ChatGPT was launched in November 2022, did you see it coming? What was your reaction when you tried it for the first time? »
Sidney Shapiro : « I think that the really cool thing about ChatGPT and other large language models is that it's very accessible. You can go to the site and you could type something in and magic happens. When I first saw the website preview of DALL-E, the ability to type something in and it generates a cat picture or a picture of an avocado walking a dog, I was like, "That's amazing. I can't imagine that technology." Since then, I've used it 10,000 times generating images for everything. Now, it's not particularly good and there's still a lot to work out, but I think it's really fantastic. Now, AI or the different branches of AI, whether it's supervised learning or unsupervised learning, has been used for a very long time, especially when you want to do things like cluster your data to find out information about different types of clients, for example, rules-based trading, AI for robotics, machine vision, machine learning, deep learning. All these different pathways have existed and exist in many of the products that we use every day. For example, your bank might tell you you're spending against your budget. Again, it's looking at the different types of data using AI. What's really changed is that not only did this new technology with transformers become very accessible, but suddenly there's no instructions, there's no technical things to learn. Everybody in the whole world can access it at the same time. I didn't see this revolution coming. Unlike many other niche technologies that really took off, people talk about it on many different levels. When I go to a conference, people are asking me about the future and what you can do right now simultaneously. Normally, there's not that much interest from the public in scientific methods or different ways that we do research. But since this is so accessible, I think it helps people overcome a lot of barriers and can produce really amazing results. »
Julien Redelsperger : « When we talk about generative AI or ChatGPT, we talk about LLMs, so large language model. Could you just explain to us what is it, how it works, and why is it so linked to generative AI? »
Sidney Shapiro : « So, when you go into a pizza store and you order a pizza, in your mind, you probably have a default order for your pizza. In Canada, the most popular pizza toppings are plain pizza and pepperoni pizza. So when you go in, you don't really think about it, that's what you ask, right? There's a strong connection between the words pizza, plain, I'd like to order, etc., that you just say by default automatically on autopilot. If you could figure out the statistical probability between all the different words you would use in a given sentence and expand that into paragraphs and context, the computer is basically looking at what are the different pathways, whether they're small words or big collections of words, that logically follow each other. Now the problem is that sometimes there's competing pathways and those competing pathways might have a totally different message, like for example, a pineapple pizza. But because we have these patterns, the computer picks one. AI is generally a black box that we're not really sure how or why it's choosing the option it chooses, but it's probably highly statistically correlated to the two different factors of what it's looking at. So we have pathways that are developed in the future. We might have explainable AI or XAI, so we could actually go and understand why the computer made the choice it made. We could also try to shape the response that the computer is giving us to incorporate other documents or factors or preferences. So maybe if you really like pineapples, it would put pineapple on a pizza, but that probably makes 50% of people very upset. So it just really depends on what exactly the computer is trying to do, but it's all about the probability in the language that already has on the language that it's trained on. So it doesn't necessarily generate new ideas and it doesn't generate big outside ideas, but it has whatever knowledge it has, it connects those pieces together and can bring them up in different ways. »
Julien Redelsperger : « And so LLM is just a statistical method to connect words together. »
Sidney Shapiro : « Exactly. So it's just patterns of words that already exist using many examples of texts and it's trained on those patterns in order to bring back results. So for example, if I had a database with hundreds of examples of people ordering pizza with peppers on it, it would become preferable over other options that are not as frequent. So we can prioritize different kinds of knowledge in order to bring back what we want to see coming out of the results. Now that's just an example of pizza, but there's many other pathways that exist with all the different kinds of language we can use to connect ideas together. »
Julien Redelsperger : « And so we can see that LLMs are becoming more accessible, like they're even running on phones. How do you see this democratizing AI for everyday users? What is it going to change? »
Sidney Shapiro : « So we're really not at the point yet of running an LLM on a phone, on your phone, like on a chip on the phone. We could connect to the internet and use a big data server to run an LLM in the cloud, which is really sitting somewhere at a data factory and being processed. I think what's really going to change is that in the future, like in the next couple of years, computer chips are being developed that actually sit on your device, are built into your fridge, are built into your microwave. And they don't know about the whole world and all the words inside of them, like ChatGPT. They just know a few very simple commands. So if you can think about it right now, you can't tell your phone, "Oh, send three messages to the past three people I spoke to," or "Cancel all my appointments." You could easily say that to a person who could then go and select all your appointments for that day and cancel them. But the phone itself doesn't yet have the ability to interact using generative AI like that, but it will be in the future. And once we have more of these chips that allow you to control the actual devices using AI, it will open up many new kinds of possibilities. Like you could talk to your car or talk to your microwave, and it will be able to do different things based on that. »
Julien Redelsperger : « Do you really think that we can have connected devices with AI? Can I ask my fridge what's in it and a couple of recipes maybe for dinner? »
Sidney Shapiro : « Definitely. We can already do that with AI in the cloud. So for example, I could take a picture of my fridge using multimodal AI and say, "Based on this picture, what do I have? What recipes can I make?" I could also tell it in text or record an audio message, or I could do all three. I could even, in the future, within the next six to 12 months, record a video and say, "Here's a video of my fridge. What could I make?" And it could tell me as well. For example, it can calculate the level of different sauces that I have in my fridge and base the recipe on that. Really advanced things. Having that AI built into your fridge means that not only is the processing done locally, which is better for privacy and better for the speed that it operates at, we're not spending as much time sending information back and forth over the internet, making it much more efficient. So that's definitely the future, having much smaller targeted models meant for specific business or other kinds of problems. So a fridge AI would be limited to things about fridges, whereas a general AI might also know about things like penguins, which your fridge might not need to know about. »
Julien Redelsperger : « And so as AI tools become easier to launch, do you think that we'll see some kind of a decline in the need for traditional developers? »
Sidney Shapiro : « I love this question. I get asked this question all the time. I would be offended by this question. People sometimes ask me, "What exactly do you do if you could be replaced by AI? AI can code. What do we need you for?" It's a really good question. I mean, where are things going? We have to look at what the state of the art is right now. In other words, what AI can produce today versus what it can do in the future. So AI right now is a tool, and a tool can help you in many different ways. For example, there's an AI called Copilot from GitHub, and it's like an autocomplete for programming. So as I'm programming and I'm typing in a really boring program, A equals one, B equals two, C equals three, the program says, "I understand the pattern you're trying to do," and it autocompletes it for me, and it does the whole alphabet. Just like on your phone, as you're typing, it knows what the next word is. The same thing in your email, the same thing, and so on. So that's like an AI example that's not generating new ideas, but it sees the patterns that I'm building and it tries to fix them for me, or try to speed it up. And usually, when I code using that technology, I save 30 to 40 percent of the time that it takes to put it together. Now generative AI, there's a lot of ideas out there, and a lot of those ideas are either wrong or they're not the right thing, or maybe people are talking on the Internet about 20 or 30 different approaches to a problem, and only one of them works really well and is right. There's definitely a difference between what AI is able to do out of the box and what people can do in terms of critical thinking. AI can recall what other people have put into the system, but it can't necessarily make up the new and the best information that it doesn't have. So because of that, people can come up with creative solutions, which computers can't do, but that's only true for today. Maybe in 10 years from now, there'll be AI that's trained on much fancier solutions with much larger datasets, and it's able to come up with better solutions. So there are some AI programmers like Devin who follow the same steps that people do, but you're not trying to create the lowest level programmer and replicate that capability. You're trying to replicate the highest level programmer, so you could tell your phone, "Create me a game," and it just builds it for you. And that technology is still 10 or 20 years away, not only because of limitations with language and the way that AIs utilize LLMs, but also because of the processing power that it would take to happen. So we've seen technologies like generative video. There's one from Google, there's one from OpenAI. But despite the fact that you could put in a sentence and get a few seconds of video back, so many things go into a video from color to motion to physics and many other things that creating a big video like 10 minutes long or two hours long is right now impossible. It would take too much processing power. So these things are possibly in the future, but there's a trade-off between how much energy does it take to produce and how much effort goes into it versus the value of the solution. So businesses right now are hesitant to replace people or processes with AI if they can't really understand the underlying value. So I would say as a programmer who builds AI, my job is probably safe for a long time. In this case scenario, AI still needs me to build more AI systems. But for most of the students that I talk to, whenever they ask this question, I encourage them to build a project entirely on AI, just follow whatever suggestion it tells you, realize that it might be terrible, and then go in this iterative loop to keep on fixing it until you get to a better solution. And I think it's important to understand what its limitations are, as well as what it can do right now. As models improve and as it has more examples of better programming, of course, the output will be better in different situations. So as a tool, I think it's amazing. It saves a huge amount of time. But to getting to the level of replacing people or completely changing what programmers do, we're still many, many years away from that happening. »
Julien Redelsperger : « So if it's not replacing people, people will still need to adapt and to adjust, of course. And so what would be the new skills or skills that we need to, that do you think will become essential for business professionals and students alike? »
Sidney Shapiro : « So I find it interesting that, I mean, I think first of all, you should become familiar with the concept of AI. I find it really interesting that people say things like, I'm going to become a prompt engineer. But what if models increase to the point where it doesn't need prompting, it could understand what you want based on the context, based on everything else you've asked, and actually get to what you're trying to do faster and better, and try to iterate with you in order to increase the quality of the output. So I think that that is definitely happening. It just means growing the model and getting bigger and bigger. I think we have this question now, we have models that are very big, and if we only made them bigger, a hundred times bigger, they'd be a hundred times better. The problem is that at size number one, we still have a lot of problems. As we scale, we have a hundred times the problems. And fixing the problems or edge cases with these models really becomes the major challenge. Like how do we actually address those issues? So I think that business people and students really need to critically think about why do we need this in the first place? What job is it fixing or what is it doing? Is it using it as an alternative? Is it using it as something else? The bottom line is that generative AI is a really massive disruptor, and it's making people think about AI in many different ways that never happened before. And suddenly we have this completely new way of looking at what are the possibilities. In Star Trek in the 1960s, people talked to the computer and the computer talked back. And now we could actually do that for real, actually happen. And I think the big difference is that not only can we do that, we could also look into the future and say, if computer speed went up so many times, these are all the new things we'd be able to do. So I think for somebody graduating today, they're going to use AI tools in the workforce. And if your only job was like writing resumes, AI can do that really well. But for all other jobs, you really have to have critical thinking of how do I utilize AI as a tool or AI that's going to be built into all my other software in order to be most effective with it. And it comes down to human traits like storytelling, like information sharing, like understanding which tool to use at which time in order to solve problems. »
Julien Redelsperger : « So you mentioned it's important to know AI. It's important to have critical thinking. What about soft skills? I heard a lot of things about soft skills, saying like, it's going to save us from AI. What do you think? »
Sidney Shapiro : « So I don't know. I mean, there's a lot of personality. If you look at Sky from OpenAI, they try to build all the soft skills into it. Personality, laughter, etc. So the real question on a fundamental level is, are those soft skills a result of programming or are those soft skills a result of being a person? And you would assume that with enough data and a big enough model that you could imitate human soft skills in a way that actually has an emotional response. So it's not going to say like, "Hal, I'm sorry, I can't do that." It would tell you a joke and try to, you know. So I don't know. I mean, I'm not sure if as far as the computer goes, can soft skills replace our working companion as a person. But I think that for people, soft skills is absolutely critical. In my area, we tend to focus a lot on hard skills. Like I know all these different programs. But ultimately, when you try to get a job, people want to know, "Are you somebody that I'm interested in working with? Can you solve the different challenges? Are you a critical thinker that's going to grab the right tools in order to put them together in a creative way?" So I think there's no replacement for those particular skills. And although, again, the way that we have AI today is maybe good at imitating them, there's still a lot of flaws. Every time this week, or in the past couple of weeks, I went to go teach a class, I asked ChatGPT for a joke that I could tell the students. I recently taught a class on web scraping. And the joke was that, although this is a class on web scraping, we're just going to scrape the surface. And the students just stared at me. And they said, "This is not a funny joke." And I said, "Okay, I mean, I'm going to try again with a different joke." And that's the problem. Like it has information, but the information out of context doesn't necessarily make sense or people find it very funny. »
Julien Redelsperger : « So we heard a lot about, of course, generative AI, ChatGPT, and so on. But we also heard a little bit about artificial general intelligence, AGI. How close do you think we are to achieving artificial general intelligence? And what could be the potential implications for businesses in the future? »
Sidney Shapiro : « So I think artificial general intelligence is very far away. It's very far away because of programming, if it's even possible. First of all, we're limited by this idea that we could put words together and any combination of words will come up with a real answer. And if you go back to when the periodic table was developed, they hypothesized that there were certain elements that would plug in later, that they knew where they were supposed to go. Later on, they found those elements, they plugged them into the right spots. So that kind of made sense. Professors are always saying, find the gap in the literature. The problem is that, if we're to get from where we are now into a computer thinking for itself, we have to go beyond language and try to find something that's even better. Meta, the makers of Facebook, had a program called Galactica that they fed articles into. And they said, okay, where's the missing gaps? Like, what's the thing we haven't researched yet? And it said things like people should eat glass, they might get superpowers. That doesn't make any sense. It's true that there's a lot of papers on eating and a lot of papers on glass, but not those two things together. And those two things together are probably not good. So they shut it down and it didn't really work out very well. So I think the idea that we could achieve AGI by using language and scaling it, unfortunately doesn't work because usually we have to find things in real life and then report on them to add to our language and what language describes. If I could read Harry Potter and then Harry Potter becomes real, then I'd be playing Quidditch right now. But unfortunately, that doesn't exist, right? So you have to look at both of those things together. So in terms of processing, AGI takes a huge amount of processing because it has to do with a lot of very complicated calculations that's driven by the computer. In other words, we're getting away from iterative steps to basically saying, what are the different possibilities? Let's pick the best one for a certain reason. And that relates to the context. So from when you're born, you have all these sensory inputs, whether it's taste, smell, touch, vision, and it's all recording in high resolution video your whole life. And that's what our training is based on. Very complicated data always coming in. And you could think about it like billions of gigabytes of all this information that we're evaluating as we're trying to make sense of the world around us. Computers right now have the equivalent, the biggest models of a 500-page book. And that 2 million context token window can tell you in the world of those 500 pages what it can do and what it thinks and so on. But to have a super intelligence that would take all human knowledge and be able to understand all of it at once to make decisions would require something multiple times bigger than what we have now. So I think in terms of the hardware issue, as far as the software issue, it's still very far away if it's possible. What I love about this question, though, is that when I go to conferences, people ask me about AGI as if it was real. So that's like saying, I'm a chef, and then people ask me about replicators on Star Trek. It's not a real thing yet. So I think that in the future, it could be. It's very unlikely, but it's possible we're going in that direction. I think as a society, we also have to ask ourselves, is this a good idea and should we be investing this? OpenAI asked for like $7 trillion to build bigger computers to try to make this happen. »
Julien Redelsperger : « But whether or not it's a good idea is not necessarily understood. And so AGI for getting better and better needs to, as you said, scrub the web, read tons of databases and news and blogs and Wikipedia articles and stuff like that. Do you think we're close to a point where there would be nothing the AI won't have read? I recently read a New York Times article that say in the future, AI will generate content to be trained on AI. It's called synthetic data. What's your take on that? »
Sidney Shapiro : « The problem is that when we get into areas that are very difficult to collect data on, I'll give you a good example. Let's say we wanted to build a website from scratch that had the location of every place in the United States and how much it costs to get internet. Right now, there's no database we could pull from. We don't have an API. Our competitors won't give us the data. So we have to call every city in North America and say, hi, what's your ISP and so on. So maybe instead of doing that, we'll build synthetic data. We'll build like what do we think the prices are based on a number of factors. And then we could use that in our model and for calculations and so on. The problem is that the internal consistency of the data may not be right. So if we're trying to do correlations and comparing data, we might be getting wrong answers. One of my colleagues went through a whole process of building datasets in ChatGPT. It took him eight hours to build this whole model so students could use it. Then he realized that all the internal consistency between the numbers are wrong. And as a result, he said, okay, I'll just build it myself in Excel. And it came out the way it's supposed to be. So I don't think that like reading every human source generates AGI. AGI goes way beyond that because it has to find the right connections between concepts and words that may not be based on things computers are good at calculating. For example, it might be based on emotion or it might be based on something else that we have perhaps we have patterns for, but I don't know if the computer is capable of making decisions outside of that framework. »
Julien Redelsperger : « So if we're going to the future, like ChatGPT was launched in 2022, we are now in 2024. We saw massive improvements. What will it look like in 2025, 2030? Where do you want it to be? And where do you think it will go? »
Sidney Shapiro : « So the biggest thing is going to happen in hardware. As we have hardware that starts expanding in new ways, that's going to create the ability to scale what we're doing now with AI, all different types of AI and build those into other systems that we use. So AI in many ways is very wasteful because I can create an Excel sheet and use it over and over again for the next 20 years and it doesn't change. I have one unit of input and thousands of units of output. But with generative AI, I would constantly be like regenerating that same sheet over and over again, paying somebody to do that or using computer resources, but it doesn't actually improve what I'm doing. So I think we lose that scale of economy with AI. There's so many places where it's useful, whether it's in health and medicine, and we can see amazing ways that we bring data together to solve real problems. Even things like video games, like as you walk around, you could have an AI talking to you with character voices, generating the video around you as you're walking around in that world and creating it just for you, like that personalized experience. And a hundred other use cases. I think the problem is that we have this amazing tool that's like a sledgehammer and we're using it for every problem, but sometimes you need a very small hammer in order to solve the problem. So people spend a lot of time building cat pictures or things like that using generative AI, and that may not be the best use of it. I think replacing or augmenting programmers and script writers and book writers and coming up with things that people are interested in reading are truly unique and don't follow the patterns of other data is still 15 or 20 years away. And the reason for that is that just becomes, not only do you need to train it on existing data, for example, read every past TV show and come up with a new one, but just by doing that, you basically get a recycled idea. Often we're looking for something new and unexpected, which again goes back to a large context. In my university, students took every Christmas movie ever made and put it into a model and then asked it generate the new best ultimate Christmas movie. And it was really terrible because it had every possible Christmas movie trope inside of it, which people said like, okay, this is way over the top, right? Maybe we have to like find a way to come up with new creative ideas. And quite often that comes down to having a human in a loop, a human who's going to augment and use AI as a tool in order to come up and bring their ideas to life. And I think it's amazing what you can do with AI in really short order. Like basically you're planning a process, you can go through AI and do it. If we go back, so I used to teach like five or six years ago, I teach a programming class and in my class I would spend most of the lecture typing things into the computer and showing the students line by line how I code. And what's amazing today is that in my class that I had yesterday, I said, let's do an example. Let's do an example about a superhero clinic. I type into the ChatGPT and it generates the database structure and schema. We could look at the diagrams, we can plan, iterate, change, update, fix, and then say, okay, great, let's build the website. I tell ChatGPT, it outputs the website, it outputs all the forms, it outputs the database, it connects it all together. And in a 15 minute demo of me using ChatGPT, copy, paste, and code, of course, instructing ChatGPT what exactly to do and fixing problems and whatever, we have a fully working website that the students can practice on, add data. We can talk about how to update apps, something that would have taken an hour and a half before. So in that three hour class, we built multiple examples of real working code and models. It's not perfect, it's not even very good, but it's the beginning point of a larger project. And if you think about what has value for a client, and if I have the ability to create a demo and then walk into a client and say, here's like a semi working version of what you want, we can then take this away and iterate and make it better and better. I think that's an amazing tool. It's an amazing capacity that we never had before. It means that we could do so much more. On a personal level, I still feel like I'm cheating because I'm getting the code generated for me and I'm plugging it in and I'm not spending an hour typing, but maybe the emphasis isn't on all the typing and showing all the students line by line. Maybe that's okay. Maybe it's okay to ask AI to help us fill in the gaps as a tool. Quite often in class, I would Google something and I still Google all the time to find out what an error means. But now if I paste that error code into an LLM, it can just come back and tell me what it means and what I should do next, which again is an amazing force multiplier. It means that we could walk away with really, really fantastic knowledge and we could start building more if we have those tools that are in a kind of automated way. But of course, no matter what you build, it's probably going to look terrible and you need a human designer to sit down and talk about colors and talk about design features and talk about what makes it special. That's not my job. That's a real person who's a designer. So I think AI is great at some things, but I think it really lacks in others. And by leveraging the possibilities of what people can do, we could build amazing things with it. »
Julien Redelsperger : « Did generative AI change anything in the way you teach things to your students? Did it change your pedagogical approach, your tools or your mindset in any way? »
Sidney Shapiro : « Definitely. So I'm on the AI committee of my university and in my class and other departments, AI filters down in many different ways. So AI affects the university as a whole in terms of policy and all the universities have this dilemma because in one department, they don't want AI because it's going to ruin whatever they're trying to do. Students are going to generate essays. In the other department, they're building AI models and they're trying to build for the future. And both of those are happening simultaneously in the same building. Within each class, there's also many things that are happening. Are you worried about students cheating? Are you worried about building the student, building one version with AI and one version themselves and then comparing them, for example, with an essay or something else? So AI is very good at doing things that already knows how to do. So it has a million examples of a resume. It can generate a resume, but it's not very good at solving problems and coming up with ideas that it's never seen before or doesn't really understand, especially large problems with many pieces. So for this semester, for the first time, I'm letting my students use AI as much as they want, solve the whole course, do everything with AI. And in my programming course, the average is still 70%, which begs the question, how's that possible if you're using ChatGPT and you're putting in the assignment and it's giving you something, how come it's not perfect? It should be 100%. And the reason why is because in my course, most of the effort that people are putting in is not necessarily the code, but it's solving a business problem, like coming up with a business case, solving a problem and making it have logical sense and supporting that by the product that you're building. So if you have a product, but there's no user and it doesn't make sense and it's too costly or something else, then you can't just generate it because that idea looks like it's complete, but it actually lacks logical sense of why you're putting it together. So I think that there has to be both. You need the critical thinking to understand why you're doing this in the first place and how it works. And the other part of it is, okay, so now that we do that, let's build a frame for it and start iterating and build each piece individually. So I always recommend with AI, start very small, make a list of the, you know, think tasks that you're trying to accomplish. For example, write an outline, understand each piece, you start working on it and ask AI to iterate, get better ideas, and then work with it to build very, very small elements at a time. There'll be much higher quality than trying to do everything at once, which usually doesn't lead to very good results. So we're right at the very beginning. And this is a highly controversial topic because so many people have different approaches to what they're trying to accomplish. For me, I want students to be aware of AI exists, it's important to know how to use it, but maybe it's also important to think for yourself and not necessarily let it tell you what to do, but vice versa. »
Julien Redelsperger : « Which challenges do you think AI will face in the future? I heard a lot of things about, you know, sustainability, about social crisis, because people are going to lose their job. Any take on that? »
Sidney Shapiro : « I think all those are true. I mean, it's very difficult to run AI, the future of AI that companies want to scale, it's going to get bigger and bigger. I think the future probably lies in a small model that's very purpose specific. So for example, you're writing emails to your customers, and it's a very small model that's trained on just that. It's efficient and economic to run because it's so small. Again, right now we're in the sledgehammer mode, so we're just trying to build bigger hammers and that's going to solve the problems. But of course, that doesn't always work. In fact, it gets so big that it makes it very difficult to work. Also in these models, as you've probably seen in the news, there's many, many problems. Like it comes out with biased information. It comes out with... So the solution for many companies is, okay, we'll build another chat bot and we're going to automate finding the problems using bots. So basically we're going to get the AI to police itself. The problem with this is that as you scale, more unexpected edge cases come up and they become more difficult, expensive, time consuming and costly to fix. So ultimately you end up in the situation that we're in the world that Google is recommending to people using their AI to put glue on pizza. And at the same time, anybody would tell you that's a bad idea, but that edge case was never asked before. So they didn't program into the system. So although we could come up with this automated scaling, it's also probably a really good idea to find out how can we build a smaller model that actually matches what we need to do in our business or for our purpose, that works very efficiently and has less problems. Recently in Canada, there was a court decision that a company is responsible for its chat bot. And because of this, people are becoming very apprehensive about just randomly putting chat bots in their business, which may have wrong instructions or offend their customers or tell them the wrong information. And as a result, we have to be very careful about how these systems are built to make sure that they're giving people the right information that's helpful, that is the right information they need at the time, based on context. »
Julien Redelsperger : « Do you think we would need more regulations? Like very recently, the European Union just passed a law called the AI Act to add guardrails on artificial intelligence. Do you think this is something that we will see more in the future in any country or territories in the world? »
Sidney Shapiro : « I think it will. I think it also has a lot to do with privacy. AI is trained on massive amounts of data. And in Europe, that was already put in place with the GDPR. So you have people who are already concerned about their data being used in general. But now companies are trying to vacuum up as much of that data as possible in order to build AI models. So looking at the two of those together, putting guardrails on AI makes sense. Programming and programming in every field is not regulated. So it becomes a challenge of how much risk you're putting into it. The Canadian government established guidelines. And they said that if it's high risk, for example, if there's a possibility of discrimination with housing, with jobs, with age, with sex, gender, etc., then your AI should be extra careful or have a human in the loop that double checks that the process that it's doing is accurate. In other words, you shouldn't have an AI firing people if it's not 100% sure and a person has verified that information. So I think as AI gets plugged into businesses more and more, these chances that something goes wrong are going to increase, which will inevitably lead to more regulation and at least oversight to make sure that AI systems are working the way they're supposed to. »
Julien Redelsperger : « Okay. And so when we talk about the future of computing, I read a lot of things on quantum computing that's supposed to revolutionize our society. What about quantum AI? Any idea what it is? Could you just explain to us a little bit if it's going to be like the future of AI? »
Sidney Shapiro : « This is my favorite question because someone recently asked me to give a talk on quantum AI, which doesn't exist. So I wasn't really sure what I should be talking about because that is not something that's real. I think the idea of having quantum computers, so we have like on a theoretical level, if we have more states and more possibilities the AI is generating, we can combine those two ideas and we could potentially have much bigger AI. The problem is that ChatGPT or other transformer languages requires a lot of stable memory, a lot of stable terms, words sitting in memory with all the tokens that link them together. And that's not really how quantum computing works. So I think in the distant future, maybe like 10 or 20 or more years from now, we have the possibility of having AI problems that are set up to be quantum problems. In other words, very large problems that have many complex subcomponents and a quantum computer can solve them quicker by sending them to the quantum computer, getting back the results and then translating that back into our context. So I think there is a possibility for something like that in the future, but we're still decades away from seeing the returns on quantum computing itself, but also on quantum computing and mixing it with other technologies like AI, which is not really there yet. »
Julien Redelsperger : « Can you give me one thing that you wish AI could do or will do in the future that's not possible to do it today? What would you expect? »
Sidney Shapiro : « So when I went, you know, I've seen recent advances and I thought it was really, really cool, the small things like schedule my next three meetings, set up alarms at different times during the week. And those are very tiny, small things, but we don't have the ability to do them yet, especially not with the critical thinking that goes into them. Like for example, I often set up classes and my classes are on Monday and Wednesday every week for 14 weeks. I don't have the ability to tell my computer, schedule my 14 classes on these two days at these two times that actually requires 20 different steps for me to do. So I think in the small things, if we have the ability to automate some of those very tedious tasks, that's going to be an amazing place that AI will revolutionize what we do. Also, you know, like I don't think that necessarily generative AI by just generating text will necessarily be useful. In fact, I think that people are going to become more aware of generated content, just like they are with spam and people see like, you know, the same sort of format or the same sort of idea and they just basically discard it. They're like, that's less important information. And I see this with my LinkedIn posts. I could generate a LinkedIn post that's like auto-generated that has the same emojis on it. And people are like, okay, not really interested in reading past the first paragraph. If I write it myself or I write it and then I ask AI for some ideas, it can do that. So recently I worked on a project with the university and it generates stories. It generates 143 trillion stories and every 60 seconds it shows you a different story. There's a display in the library for AI month in June that shows you all the possible stories. I was like, okay, that's interesting. But how many slices of pizza would it be if you took 143 trillion slices of pizza and stack them on top of each other, how far would that go? And that was the perfect question for AI to answer me. Like it said, okay, based on the size of the pizza, the thickness, etc. And it came back and said 1.5 million miles. So great. I wrote a pizza saying this program is like 1.5 million miles of pizza, an easy thing to do. So I think that, that being curious and looking at how the technology is developing in the future and where it's going means that there's going to be really amazing things happen. Transformer technology using language itself as a basis, maybe isn't going to be the panacea or the end all of what AI can do. Looking at new technologies that are coming out now, translating between languages, using AI that you could talk in any language in the world and understand it in another. Having translation that's based on not just words, but on the context, like the paragraph is amazing. And it means that not only be able to communicate, people will be able to work safer, will understand our data better, it'll be more efficient. And there's a lot of reasons why AI is doing really fantastic things, whether they're happening like in public or whether they're happening behind the scenes as people develop them. But I think from both, there's going to be really, really amazing advances using this technology that we're just starting to scratch the surface. And we really haven't got to the point where we're building things that like this is the last iteration of what we're building. We're right at the very, very beginning. »
Julien Redelsperger : « So you don't seem particularly worried about the future of AI. You seem pretty excited about it. »
Sidney Shapiro : « I think so. I'm not worried at all. I think it's very exciting. And every day new things come out. I have a newsletter that every day sends me four new things in AI. And the interesting thing is that after a year and a half, there's still four new things coming out every single day, hundreds of papers, people are talking about it. People are using AI in unexpected and different ways. I recently met a professor who said that their students build math proof problems in a math course and they just take the proofs that they figure out, which ChatGPT can't do. It doesn't have processing power behind it. It just can string the language together. And the student says, here's my math proofs that I proved that I figured out, write the thesis around it. And it writes the descriptions of the proofs and it puts it together. And in their field, they accept that because again, you can't use generative AI to get the problems that they're doing or build the mathematical proofs, but you can use ChatGPT to explain what's going on in layman's terms, which is amazing because that's what the thesis is supposed to be. So you have many different fields that are embracing AI and wondering how they're going to work. And right now, business adoption has been very low in my field because people are still saying, where is this going to go? What exactly does technology do? How do we put it together to make it work? And I think that as people get more comfortable with mature AI and they start seeing really interesting use cases to plug it in, then we're going to see a lot of scaling and people are going to start building into programs to help scale what they're able to do. »
Julien Redelsperger : « But do you understand that sometimes some people are okay? I mean, it's okay for them to be worried about. I'm thinking about, you know, translator, I'm thinking about copywriters, I'm thinking about the strike in Hollywood last year, for example. Do you understand that sometimes in some specific context and situation, AI could be a truly game changer that changes everything? »
Sidney Shapiro : « I think it can do some pretty amazing things. I haven't seen the amazing game changer yet. And the reason for that is that we're not watching TV made by AI. We're not reading books that are generated by AI yet. We're not playing video games that are made by AI. And we're not doing programs that are better than people can do them, again, by AI. So I think we could see these things in the future and they might be possible. They might be possible leveraging what we have now in the future. And of course, people are worried about them because that's going to completely change the nature of their work. But I think that if people are using AI as a tool and we're building in that consideration that maybe AI shouldn't be out to, you know, deliver shareholder value by completely replacing people but instead augmenting what they can do, it's going to lead to some pretty fantastic results for most people. I think even for the person who's afraid of their job as a writer being taken out, imagine that writer as they're typing on their computer, we can generate storyboards of the pictures that are doing using generative AI. That technology already exists and people are actually using it in industry. So yes, it's generating, it's not necessarily competing with them, it's helping them as they start working in their job using these technologies. »
Julien Redelsperger : « Cool. Perfect. Well, thank you so much, Sidney. I really appreciate your time here. So at the end of each episode, the guests must answer a question posed by the previous guest. After that, you'll have the opportunity to ask a question for the next guest. Are you ready? »
Sidney Shapiro : « Ready. »
Julien Redelsperger : « Perfect. So here's your question, courtesy of Luca Zambello, who is CEO and founder of Jurny. It's an AI-powered solution to help property managers. You can listen to his question right now. »
Luca Zambello : « What is the most significant challenge you have faced in your career and how do you overcome it? »
Sidney Shapiro : « Well, I think I have one obvious answer. So I would say that the most significant challenge is completing my PhD. I recently had coffee with someone in industry that we were talking about a project and he said, "You're amazing. I can't believe how smart you are. You're a genius." And I said, "You know what? I'm really not. I'm really not. I'm just very determined." And being in a PhD that takes five years and people say, "You can't do it and it's not working out." And despite that, you push through and you keep on writing and you keep on researching and put it together. You know, eventually you get to the point where you say, "I can accomplish anything and no matter what happens, I'll get to the end." And I think that was by far the biggest challenge I've ever faced in my life. I would never do another PhD. I know some people do that. It's a terrible idea. But really the idea is coming up with your beginning question and then seeing how it evolves from there. You don't really know very much. You learn a lot along the way. And I think if I went back today and completed that PhD again, it would take me a year. And I often think about what was I doing for the other four years? I learned so much, whether it was like theory or code or the work that I put into it. And that model of what I did led me to where I am today. Like I'm a professor of business analytics and I did my whole thesis on analyzing data. It was different kinds of data. I did social network analytics, but it taught me determination, tenacity. I had lots of support from my friends and colleagues to get through this process. And that was really the most and biggest and most complicated thing I ever accomplished. So now with AI, I often think, what would my thesis look like if I had AI to come up with ideas and regenerate sentences? It would be very, very different. And for my students today, just because they're generating or they're asking AI, it doesn't necessarily mean the ideas are good or they make sense or they're well written. So I think we live in a different world. And a part of that is really thinking about how do we leverage AI as a tool in order to get where we're going? »
Julien Redelsperger : « So do you think a PhD student today would think differently than a PhD student like five years ago? »
Sidney Shapiro : « I think there's just a reality that AI exists and it's out there and everybody's talking about it. So whether or not you're using it, whether or not you're specifically putting that into your thesis or building it in, it exists and we live in a world with it in every discipline. So I think we really have to acknowledge that and say, if this is a reality, how do we work with it? When you're at a conference with colleagues, every conference I go to now, even professional ones, everyone's talking about AI in different contexts. So they're trying to understand, is this a threat to me? How do I build this into my practice? Does it make sense? Do I ignore it? Do I just wait five more years and see what happens? And those answers are really going to dictate where we go and how academia looks like over the next few years. »
Julien Redelsperger : « Cool, cool. Perfect. Well, thank you so much for that. So now what question would you like to pose for the next guest? »
Sidney Shapiro : « Would you be comfortable scaling your AI use if it meant sharing data with external companies for training purposes? »
Julien Redelsperger : « Perfect. It's very well correlated to the news. »
Sidney Shapiro : « Exactly. »
Julien Redelsperger : « Cool. Perfect. Well, thank you so much, Sidney. It's been an absolute pleasure speaking with you today. Thank you for joining me. »
Sidney Shapiro : « Thank you very much. »
This transcription was created using an artificial intelligence tool. It may not be 100% accurate to the original content and may contain errors and approximations.